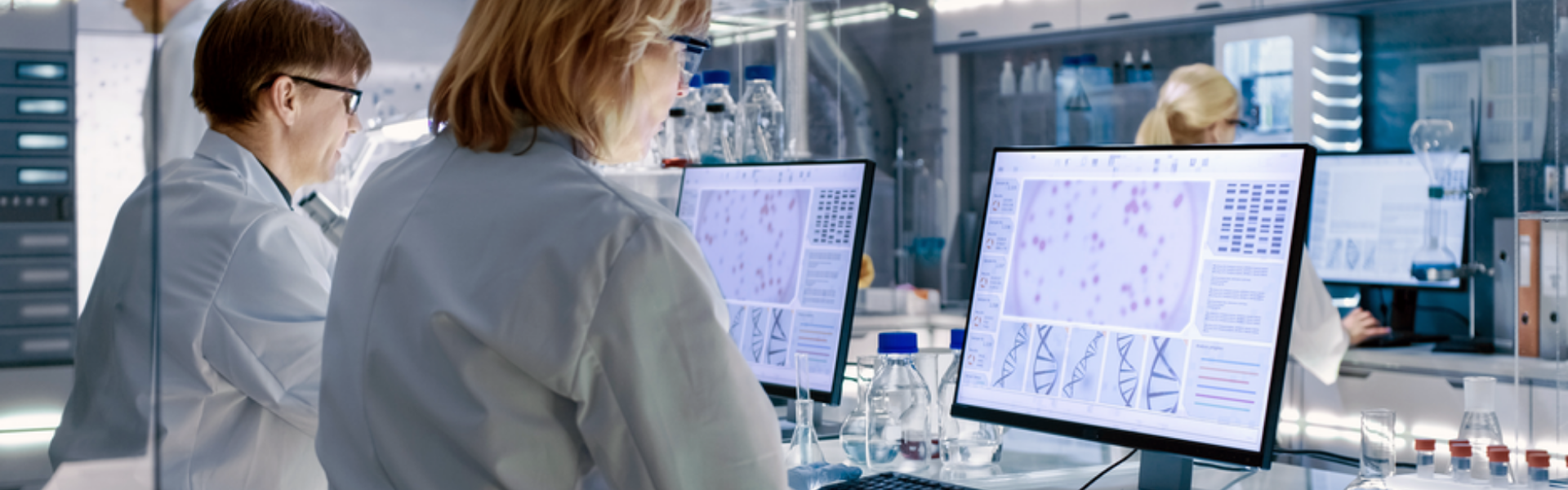
Better care through better data analytics? Here's how
July 8, 2021 | By Laura MollFashionably late to the party, sophisticated analytics are identifying efficiency and predictability in the business of health care. Powered by the continuing digitization of patient, provider and payer data, analytic engines are uncovering critical insights to improve both outcomes and the financial bottom line. The question is no longer whether data-driven insights can address some of the largest pain points for the industry but rather, given the success of trailblazing initiatives, how far can we go?
01
Mitigating fraud, waste and abuse
Fraud, waste and abuse in health care claims costs health insurers (payers) an estimated $300 billion in overpayments each year. To make matters worse, when a problematic claim is flagged (generally less than 10% of the time), it is usually uncovered after the payer has reimbursed the doctor or hospital (provider). Leveraging artificial intelligence up front, as claims are submitted, has now been shown to significantly reduce the risk of overpayment by flagging problematic claims earlier in the process.
The evidence for this high-potential opportunity comes from a recent Mastercard initiative conducted in partnership with a health care payment integrity vendor. Under the assumption that current fraud-detecting AI solutions for consumer and enterprise payments could serve as a platform, Mastercard and its partner applied a regimen of supervised and unsupervised learning methodologies to create a new, continuously evolving model for identifying out-of-pattern data in health care claims. Focusing on anomalies, this new model was able to quickly identify 2,700 high-risk providers and $240 million in potential savings for the payment integrity provider.
Beyond the proven results, the value of the model in identifying potential fraud was further enhanced by its deployment “pre-adjudication,” i.e., before the payer would reimburse the provider for services. This produces benefits both in the near term and further downstream, when it can be much more costly to unwind. This early intervention approach effectively shifts the paradigm from “pay and chase” to “detect and save.”
02
Optimizing value-based care
In a bid to control costs and improve outcomes, the U.S. health care system is moving toward a value-based care model. Under value-based care, providers are reimbursed not on the administration of specific tests or therapies, but based on the overall outcome for the patient. The incentive is to maximize health outcomes and minimize the use of costly but ultimately unnecessary or ineffective treatments.
Value-based care operates on a carrot-and-stick model. If patient outcomes are good, providers earn incentives. If outcomes are poor, providers can be subject to readmission penalties. Therefore, providers have strong motivation to identify the optimal treatment protocols that minimize cost and maximize patient outcomes.
One way to do this is by running data experiments on prior outcomes to develop a predictive solution. For example, running test vs. control analyses to compare the effects of alternate treatment protocols can identify specific courses of action that will lead to fewer days in the hospital and/or fewer readmissions. Predictive analytics can also identify the patients most likely to respond well to care pathway A versus care pathway B, and can provide insight into which particular tactics within a care pathway are likely to have the greatest overall impact.
Here again, the evidence comes from a Mastercard initiative launched in partnership with a large regional health system. In one example, the provider was able to design an achievable set of protocols for congestive heart failure that improved all measured metrics, including reductions in length of hospital stay and readmissions. In a second example, the data revealed the importance of specific protocols to be followed by patients recovering from a specific class of surgeries in reducing length of stay.
Once a hospital optimizes treatment protocols in one location, replicating those methods across a health system can yield substantial improvements in patient outcomes as well as operational efficiencies.
03
Incentivizing behavior
Providers can also use data-driven insights to identify and encourage behaviors correlated with improved outcomes. As in the case above, post-treatment behaviors are often correlated with better outcomes. But identifying the behavior is not enough — the patient must adhere to whatever regimen is prescribed (or refrain from others).
In commerce, loyalty solutions can be tremendously effective at encouraging customer interest, retention and repeat visits. It stands to reason these same incentive-based solutions could be targeted toward health behaviors, too. For example, motivating a hip replacement patient to complete the prescribed rehab exercises, attend physical therapy appointments and take medications will both improve the patient’s prognosis and produce savings for all parties over the course of the recovery. Motivating a pregnant woman to attend prenatal appointments, take prenatal vitamins and follow a healthy diet improves both the pregnancy and the long-term health of the child.
Beyond the right incentive, these data-driven solutions can determine which mix of tactics (e.g., reminders, educational messaging, positive reinforcement, rewards — with or without actual monetary value, etc.) will lead to better adherence for any given patient. By understanding how and where to deploy incentives, and how to achieve a return on the investment, health care organizations can improve engagement, leading to better overall outcomes for patients and lower costs all around.
04
Right-pricing treatments
Analytics are also being used to address health care affordability. Given the high costs associated with care and the unpredictable nature of injuries or ailments, health care expenses can create significant financial hardship for consumers. Because there are moral considerations involved with aggressive collections approaches in health care, providers are often challenged to recover fees for services rendered. But most patients would pay their bills if they could.
One idea is to leverage the potential of open banking capabilities (i.e., permission-based access to a patient’s financial data), paired with front-end analytics that assess a patient’s ability to pay. Armed with these tools, providers can offer individualized “right pricing” that reflects a patient’s unique circumstances. This custom approach meets patients where they are with charges that more closely reflect what they can afford. In combination with tailored payment plans or financing, this methodology has proven to yield an increase in revenue from patient receivables.
05
A force multiplier for health care
As the trend toward digitization in health care continues, providers, payers and patients will encounter more and more opportunities to employ data and a variety of analytic tools to benefit both health and financial outcomes. Along the way, fostering patient trust in the care and use of data will be critical to the long-term acceptance and adoption of analytic programs. How will data be collected, stored, used and shared? The potential for unintended consequences is similarly felt by patients, shareholders, medical review boards and regulators alike.
But with the appropriate measures in place, a broader reliance on data and analytics holds enormous potential to overhaul and revolutionize the health care industry. By promoting more effective treatment plans, encouraging healthy behavior, and encouraging more efficient delivery of services data and analytics have the potential to be a force multiplier for health care.
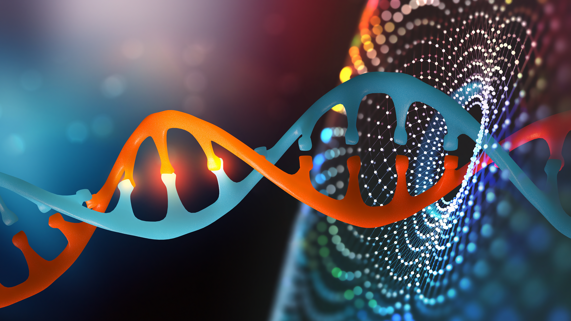